Last Updated on June 2, 2024 by Daniele Lima
Integrating technology and planning to combat fraud
In the dynamic and interconnected landscape of modern business, fraud detection has become an undeniable priority for organizations looking to protect their assets and maintain the integrity of their operations. As we move into a digital age, traditional fraud prevention strategies are being challenged and often surpassed by the cunning and sophistication of fraudsters. In this context, there is an immediate need to explore innovative strategies and advanced technologies to detect and prevent illicit activities.
This article proposes an in-depth analysis of strategies emerging in the field of detecting fraud, highlighting not only traditional threats but also new nuances that emerge in a constantly evolving digital environment. From analyzing behavior patterns to implementing artificial intelligence and machine learning, we’ll examine the latest approaches that are shaping the detecting fraud landscape and empowering organizations to tackle increasingly complex challenges.
Table of Contents
What is fraud detection and prevention?
Fraud detection and prevention are essential for protecting organizations from potential fraudulent activities. Implementing effective strategies to detect and prevent fraud helps companies safeguard their assets and reputation in today’s complex business environment. Detecting fraud requires actively monitoring financial transactions, employee conduct, and warning signs that could signal deceptive behavior.
Conversely, preventing fraud involves establishing and enforcing strong policies, procedures, and internal controls to reduce the likelihood of fraudulent incidents. By promoting a culture of vigilance and honesty, businesses can strengthen their defenses against fraud and build trust with stakeholders.
Fraud Detection (fraud detection models):
- Fraud detect refers to identifying fraudulent activities after they occur. This involves monitoring transactions, behaviors, and data patterns for activity that could indicate fraud. Detection can occur in real-time, immediately after a transaction is performed, or through retrospective analysis, examining historical data to identify suspicious patterns.
- Fraud Prevention:
- Fraud prevention, on the other hand, involves implementing proactive measures and controls to prevent fraudulent activities from occurring in the first place. This includes adopting security policies, implementing advanced technologies, and educating users and customers about safe practices, among other preventative strategies.
Both detection and prevention approaches are crucial to a robust fraud management program. Prevention seeks to create barriers and make it difficult to carry out fraudulent activities, while detection comes into play to quickly identify and respond to suspicious events that have managed to overcome preventive measures.
Examples of Fraud Detect and Prevention Measures:
- Detection:
- Real-time monitoring of financial transactions.
- Analysis of user behavior patterns.
- Use of machine learning algorithms to identify anomalies.
- Automatic alerts for suspicious transactions.
- Judicial analysis of data after detection of possible fraud.
- Prevention:
- Multifactor authentication for sensitive access.
- Implementation of firewalls and malware protection systems.
- Educating users about safe practices, such as not sharing passwords.
- Regularly updating systems and software to fix vulnerabilities.
- Geographic restrictions for online transactions, when applicable.
Which Is A Tool Can Be Used To Automate The Fraud Detection Process?
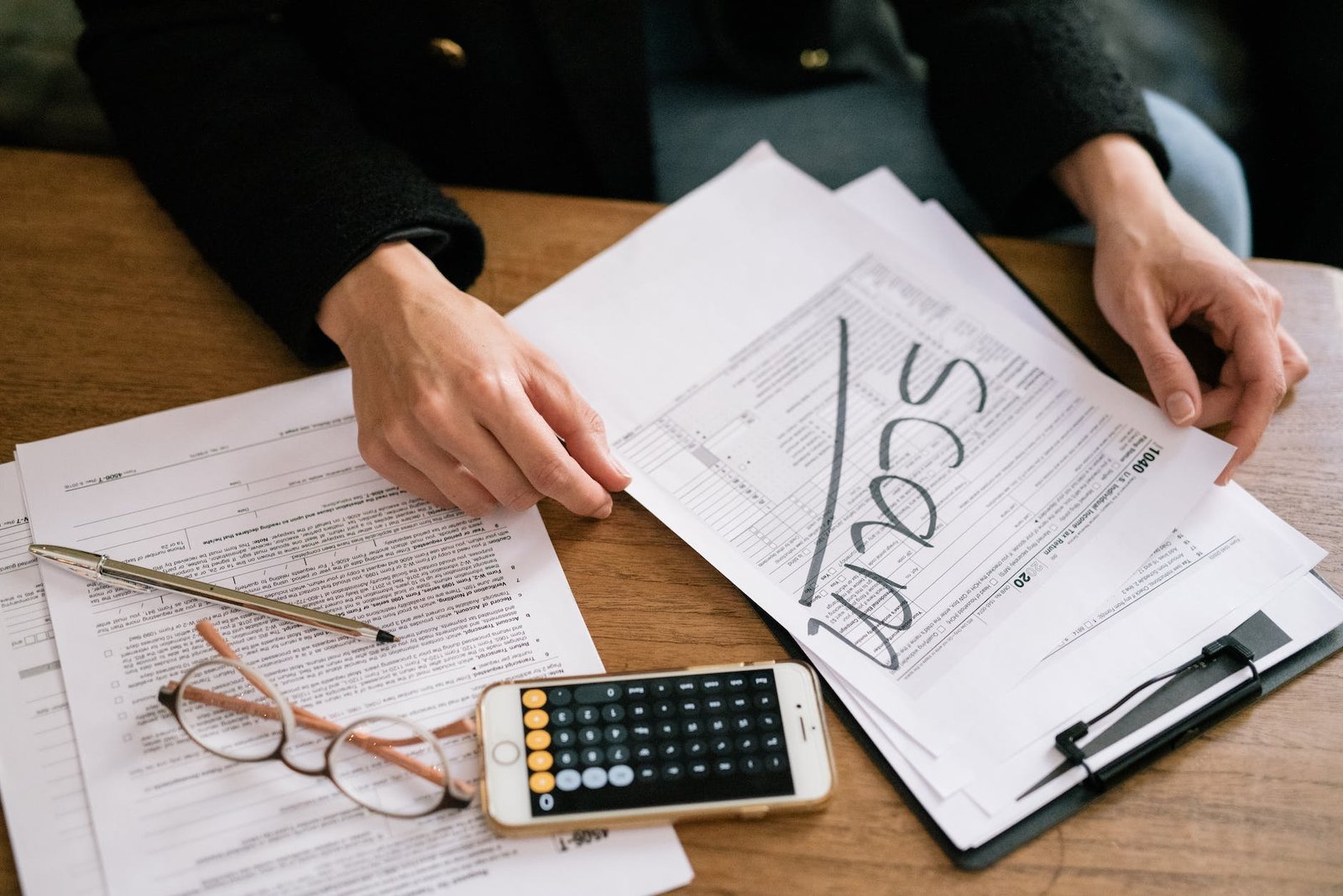
A widely used tool for automating the detecting fraud process is data analytics and machine learning software. Among these tools, the following types stand out:
Artificial Intelligence (AI) and Machine Learning (ML) Platforms (AI fraud detection):
This revolutionary tool is designed to use cutting-edge machine learning to efficiently process and analyze large amounts of data. They can use the power of artificial intelligence to see complex patterns that are indisputable and potentially objectionable.
These tools allow complex data to be analyzed quickly and identify correlations and patterns, providing valuable information that would otherwise be overlooked. Leveraging advanced analytics and predictive modeling, these advanced solutions help organizations improve decision-making, identify vulnerabilities, and strengthen security measures.
Predictive Analysis Tools ( fraud detection techniques):
They use statistical models and algorithms to predict future behavior, allowing them to anticipate possible fraudulent activities based on historical patterns.
Anomaly Detection Systems ( fraud detection systems):
They identify significant deviations from normal behavior patterns, warning about atypical activities that may indicate fraud.
Social Media Analysis Platforms:
They analyze connections between entities, such as customers, transactions, or accounts, to identify patterns of behavior that may indicate fraudulent network activity.
Data Mining Tools:
Extract valuable insights from large data sets and identify correlations and patterns that may indicate fraud.
Real-time Fraud Management System (real time fraud detection ):
Monitors transactions in real-time using rules and algorithms to identify and stop fraudulent activity before it causes harm.
Behavioral analysis platform:
Evaluates typical user, customer, or device behavior to identify anomalies based on changing patterns that may indicate fraudulent activity.
These tools, often integrated into broader risk management systems, are essential for promptly detecting fraud in complex and dynamic environments, providing organizations with an efficient and effective way to protect their assets and maintain the integrity of their operations.
Why Would A Financial Institution Automate Their Fraud Detection Process?
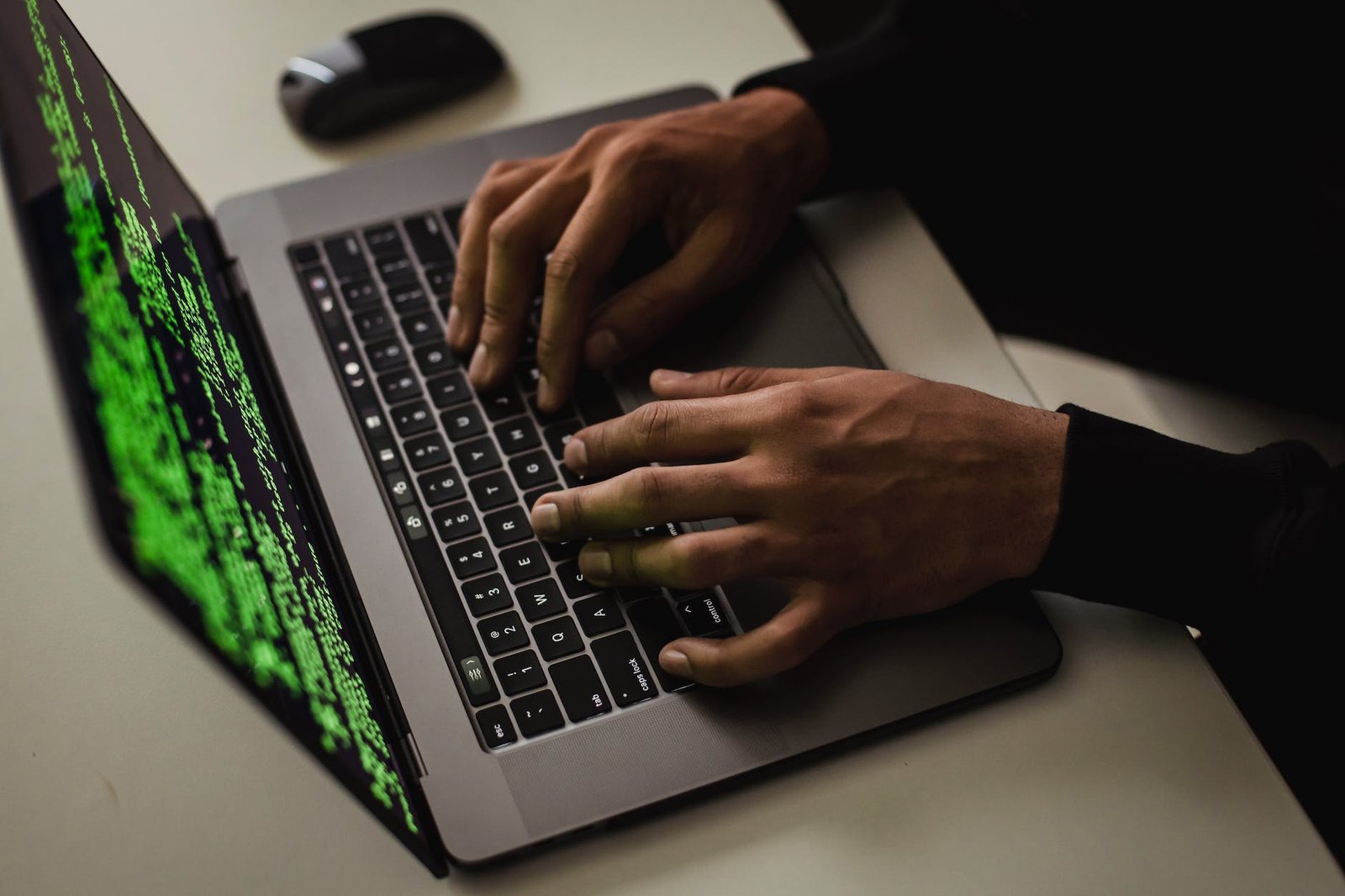
There are a number of key reasons why a financial institution might choose to automate detecting fraud processes, reasons that reflect the challenges and demands of the modern financial environment. Some of these reasons include:
Speed and efficiency: Automation allows the immediate processing of large amounts of data. This is important to quickly identify and respond to fraudulent activities, minimizing the financial and reputational impact.
Scalability: As the number of transactions continues to grow and threats continue to evolve, automation allows the registration process to scale to handle increasing volumes without compromising efficiency.
Accuracy and consistency: Machine learning algorithms and other automated techniques can provide more accurate and consistent analysis of user behavior and trading patterns. This reduces the need for manual methods prone to human error and inconsistencies.
Adapting to new fraud patterns: As fraud methods evolve, automated systems can adapt and update more quickly than manual processes. This allows financial institutions to readily adapt to new threats.
Reduce operating costs: Automating detecting fraud processes can reduce costs associated with manual work. This is especially important when dealing with large amounts of data and transactions.
Reduced reputation risk: Effective and timely detection of fraud helps maintain customer trust and prevents them from becoming victims of fraudulent activity. The reputation of a financial institution is directly related to its ability to protect the interests of its customers.
Regulatory Compliance: Many regulations require financial institutions to implement rigorous fraud prevention and detection procedures. Automation makes it easier to comply with these rules, ensuring robust and controllable processes.
Big Data Analysis: As financial institutions process large sets of data, automation is critical to effectively extract meaningful information, identifying patterns and anomalies that could indicate fraudulent activity.
By combining advanced technologies such as artificial intelligence and machine learning, financial institutions can significantly strengthen defenses against fraud, delivering benefits in terms of financial security and customer trust.
How does credit card fraud detection work?
Credit card detecting fraud is a complex process that involves constant monitoring of transactions to identify suspicious patterns or unusual activity. This is the general process for detecting credit card fraud:
Real-time monitoring:
Financial institutions monitor transactions in real-time. This includes in-store purchases, online transactions, ATM withdrawals, and other types of transactions. Cardholder information:
Create a profile for each cardholder based on their usual transaction history. This includes spending habits, frequented locations, typical transaction times, etc.
Anomaly detection algorithm:
Anomaly detection algorithms are used to identify transactions that differ significantly from typical cardholder behavior. This includes unusual quantities, non-standard business locations, and activities outside normal business hours.
Location:
Using geolocation can help verify whether the location of the transaction matches cardholder behavior patterns. Transactions in remote locations that do not match history may trigger alerts.
Machine Learning and Artificial Intelligence (machine learning for fraud detection):
Machine learning systems analyze large amounts of data to identify complex patterns and fraud. These systems can constantly adapt, learn new data, and evolve to detect new threats.
Risk filters and fraud scores:
Each business is evaluated based on the degree of risk. This score is determined by several factors, including transaction value, location, frequency, and cardholder service history. Transactions with high scores may be flagged for further analysis.
Custom logging rules:
Financial institutions can establish specific rules to detect known fraud patterns. For example, blocking transactions in certain high-risk areas or limiting the number of consecutive transactions in a short period of time.
Messaging and communicating with cardholders:
If a transaction is considered suspicious, cardholders may receive text, email, or mobile app notifications prompting them to confirm or alert them to suspicious activity. If in doubt, take immediate action:
If a highly suspicious transaction occurs, the bank can take immediate action by freezing the card and contacting the cardholder to confirm the legitimacy of the transaction. This ongoing monitoring and analysis process is critical to ensuring the effective detection of credit card fraud and protecting cardholders and financial institutions from fraudulent activity.
Conclusion: Unlocking the future of financial security
We conclude that effective detection is not just a reaction to past events, but also a vivid reflection of the evolution of the financial world. By implementing and integrating innovative strategies, financial institutions not only protect their customers and assets but also actively shape the story of a sustainable, dynamic, and adaptive financial industry. This makes it gain credibility in the market due to the security measures. It is a challenge and an opportunity that will define the future of detecting fraud, an ongoing journey towards unwavering security and trust, in the world of digital financial transactions.
FAQ
What is the most common way to detect fraud?
The most common way to detect fraud is through real-time monitoring of transactions. Financial institutions constantly monitor transactions for any unusual patterns or suspicious activities. They employ algorithms and machine learning techniques to analyze large volumes of data, identifying anomalies that deviate from typical user behavior or transaction patterns. Additionally, geolocation tracking helps verify transaction locations, flagging those that are inconsistent with the cardholder’s usual behavior.
What is the basis of detecting fraud?
Detecting fraud is based on analyzing transactional data and user behavior. Financial institutions create profiles for each cardholder, considering their typical spending habits, transaction locations, and timing. Algorithms then detect anomalies by comparing current transactions to these established patterns. Machine learning and artificial intelligence play vital roles in identifying complex fraud patterns and adapting to new threats.
What are the rules for detecting fraud?
Rules for detecting fraud include real-time transaction monitoring, analysis of user behavior patterns, use of machine learning algorithms to identify anomalies, geolocation verification, risk scoring, and personalized detecting rules. These rules help financial institutions identify suspicious activities promptly and take appropriate action to mitigate potential risks.
What are the steps in identifying fraud?
Identifying fraud involves several steps, including real-time monitoring, analyzing transaction data, profiling cardholders, employing machine learning algorithms, geolocation verification, risk scoring, setting personalized detecting fraud rules, and communicating with cardholders to confirm or flag suspicious activities. These steps ensure a proactive approach to detecting fraud, enabling financial institutions to protect their customers and assets effectively.
Leave a Reply